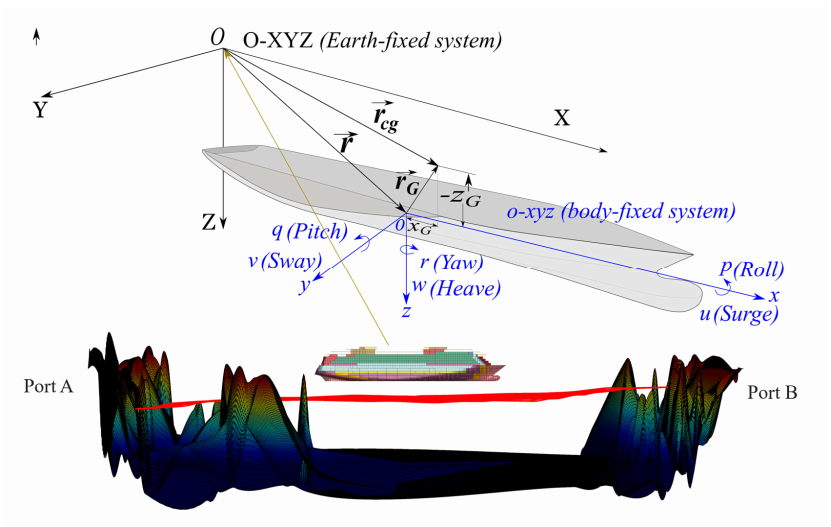
COMPARISON & EVALUATION OF LEARNING CAPABILITIES OF DEEP LEARNING METHODS FOR PREDICTING SHIP MOTIONS
Mingyang Zhang a, Cong Liu a, Pentti Kujala b, Spyros Hirdaris c
a Department of Mechanical Engineering, Marine Technology Group, Aalto University, Espoo, Finland
b School of Engineering, Aalto University, Espoo, Finland
Tallinn University of Technology, Estonian Maritime Academy, Tallinn, Estonia
c American Bureau of Shipping, Global Ship Systems Centre, Athens, Greece
Presented at the 15th International Marine Design Conference – June 2024
ABSTRACT
The development of intelligent ship control systems in real-world conditions relies heavily on the accurate identification and prediction of ship seakeeping and maneuvering trajectories. In this study, we comprehensively evaluate a selection of deep learning methods to assess their learning capabilities in terms of idealizing ship motion behavior in realistic operational environments. To recover real conditions, we utilize historical Automatic Identification System (AIS) data and a time domain 6 Degree of Freedom (6-DoF) grounding dynamics model to generate ship motion sequences for a Ro-Ro passenger ship operating in the Gulf of Finland. Via a rigorous evaluation process, we validate the performance of these methods using extensive data streams. The analysis includes the identification and estimation of uncertainties between two ports. The paper demonstrates the proficiency of the selected deep learning methods in capturing ship maneuvering features, their potential use in the design of ship control and intelligent decision support systems.