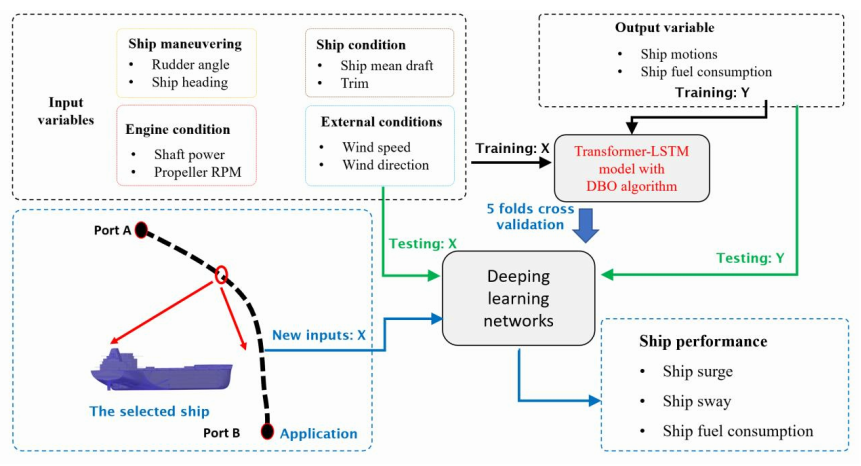
AI MODEL FOR THE PREDICTION OF SHIP MOTIONS AND FUEL CONSUMPTION OF A KAMSARMAX BULK CARRIER
Mingyang Zhang a, Pentti Kujala b, Spyros Hirdaris c, Nikolaos Tsoulakos d
a Department of Mechanical Engineering, Marine Technology Group, Aalto University, Espoo, Finland
b School of Engineering, Aalto University, Espoo, Finland
Tallinn University of Technology, Estonian Maritime Academy, Tallinn, Estonia
c American Bureau of Shipping, Global Ship Systems Centre, Athens, Greece
d Laskaridis Shipping Co. Ltd
Presented at the Global Conference in Naval Architecture and Ocean Engineering, Southamton (UK) – November 2024
ABSTRACT
This paper introduces an Artificial Intelligence (AI) model designed to predict ship motions and fuel consumption for a Kamsarmax Bulk Carrier under varying wind conditions. The dataset employed encompasses navigation and wind data, ship operational conditions, and engine data from a Kamsarmax Bulk Carrier managed by Laskaridis Shipping Co. Ltd. over an one-year period. Initially, a decision tree (DT) methodology is applied to analyse extensive big data records including data streams of relevance to ship manoeuvring and energy systems performance. A hybrid deep learning model is utilized to capture the intricate dynamics of ship systems and to develop an AI-based surrogate model, by combining Long Short-Term Memory (LSTM) and Transformer models. The study concludes that the developed AI-based surrogate model has the potential accurately predict ship motions and fuel consumption under varying environmental conditions. Subject to further validation, the model could serve as a valuable tool for ship operators.