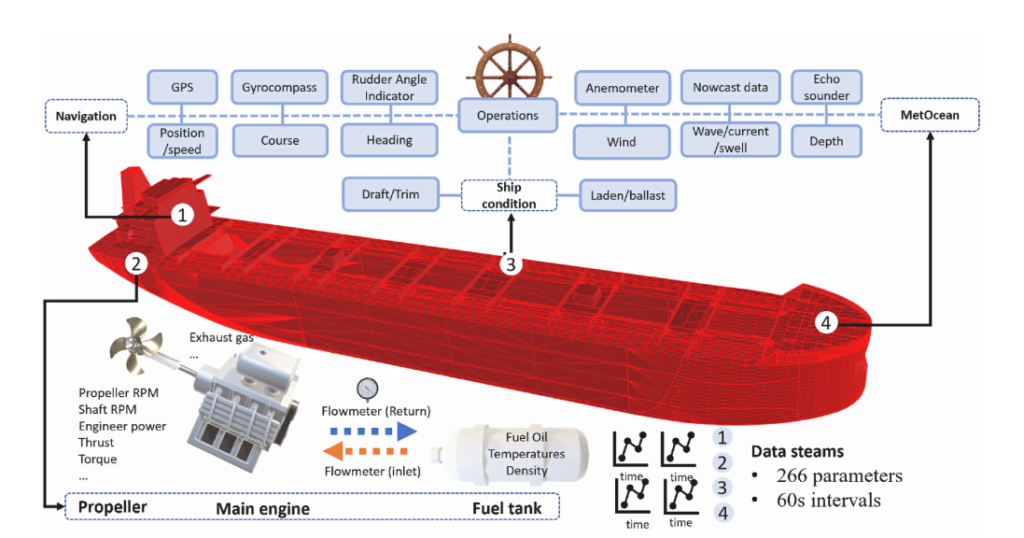
DEEP LEARNING METHOD FOR PREDICTION OF SHIP FUEL CONSUMPTION
Mingyang Zhang, Pentti Kujala, Spyros Hirdaris, Aalto University, Finland
Nikolaos Tsoulakos, Laskaridis Shipping Co. Ltd
ABSTRACT
In recent years, the European Commission and the International Maritime Organization (IMO) implemented various operational measures and policies to reduce ship fuel consumption and related emissions. The effectiveness of these measures relies upon developing accurate predictive models encompassing the influence of real operational conditions. This paper presents a deep learning method for the prediction of ship fuel consumption. The method utilizes big data analytics from sensors, voyage reporting and hydrometeorological data, comprising of 266 variables made available following sea trials of a Kamsarmax bulk carrier of Laskaridis Shipping Co. Ltd. A variable importance estimation model using a Decision Tree (DT) is used to understand the underlying relationships in the available dataset. Consequently, a deep learning model is developed to understand the influence of sailing speed, heading, displacement/draft, trim, weather, sea conditions, etc. on ship fuel consumption (SFC). This is achieved by incorporating attention mechanism into Bi-directional Long Short-Term Memory (Bi-LSTM) network. The potential of the new method is demonstrated by training data streams corresponding to real ship fuel consumption rates as well as internal and external operational conditions. A comprehensive comparison with existing methods indicates that the Bi-LSTM with attention mechanism presents the best ft when using high frequency data. It is concluded that subject to further testing and validation the method could be used for the development of decision support systems for monitoring environmentally sustainable ship operations.